The aim of this project is the performance of Predictive Maintenance (PdM) tasks in a miniaturised gear pump. This consists of first, identifying possible faults (diagnosis) throughout the lifetime of the pump and, second, predicting the remaining useful life (prognosis) of the system. The miniaturised gear pump is used as part of a complex printing machine used to print sophisticated labels. This international project has been proposed by F-Lab, Milano, in collaboration with Prof. Ferdinando Auricchio (Università di Pavia) and Prof. Antonio J. Gil (Swansea University, UK).
As a first step, we performed Computational Fluid Dynamics (CFD) analysis for the gear pump to generate high fidelity data which can then be used to feed Artificial Intelligence (AI) algorithms. The CFD analysis is carried out using PumpLinx® software, which relies on the use of an unstructured cell centred Finite Volume method to solve the set of underlying fluid cavitation conservation equations [1].
In a second step of this project, we combine tailor-made Artificial Intelligence (AI) algorithms, also known as Machine Learning algorithms, with the high-fidelity data to conduct PdM. Additional data is manufactured through random perturbation of the high-fidelity results, used to further fed the Machine Learning engine.
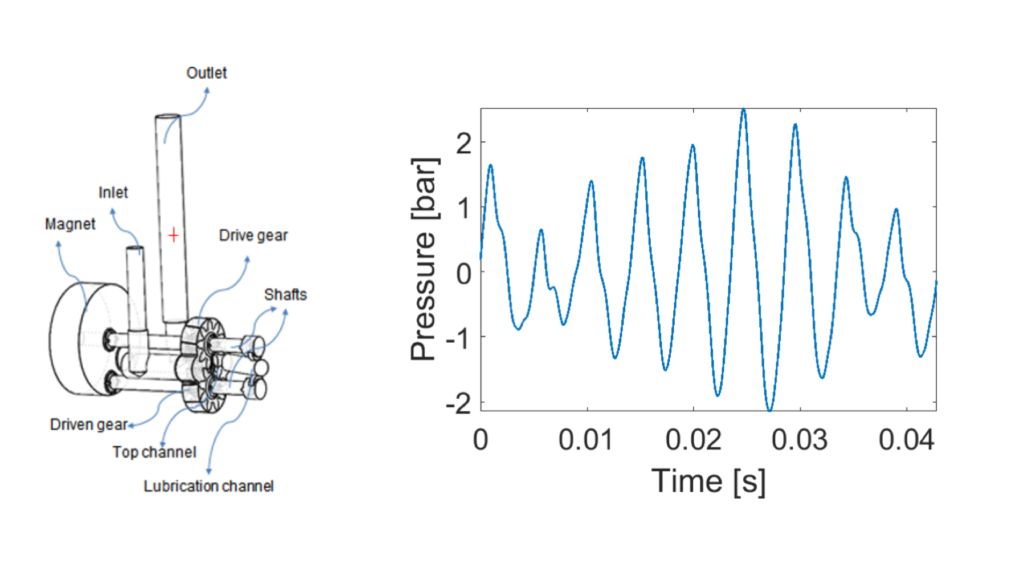
Fig. 1: Geometry of the gear pump (left), discharge pressure (+) of the pump for 1 revolution [0-0.0429 s] (right).
To differentiate between healthy and faulty situations, we introduce defects/faults and/or parametric variations within the pump, in order to establish a sample spectrum of realistic scenarios. We extract appropriate features from the data signal using Auto Regressive models and/or Wavelet Transforms.
As far as AI is concerned, we explore Support Vector Machine (SVM) classification and regression methods to perform diagnosis and prognosis, respectively. SVM is a classification machine learning algorithm, which learns from the training data set and classifies the test data with high accuracy. With the availability of life time degradation data sets, prognosis can also be performed. With this in mind, an exponential degradation model [2] will be considered to predict the remaining useful life of the equipment. As a final step, alternative AI algorithms will be considered in order to explore their reliability and robustness [3].
References
[1] Ding, H., Visser, F.C., Jiang, Y. and Furmanczyk, M., 2011. Demonstration and validation of a 3D CFD simulation tool predicting pump performance and cavitation for industrial applications. Journal of fluids engineering, 133(1), p.011101
[2] https://it.mathworks.com/help/predmaint/ref/exponentialdegradationmodel.html
[3] Saha, B., Goebel, K. and Christophersen, J., 2009. Comparison of prognostic algorithms for estimating remaining useful life of batteries. Transactions of the Institute of Measurement and Control, 31(3-4), pp.293-308
July 8th, 2019